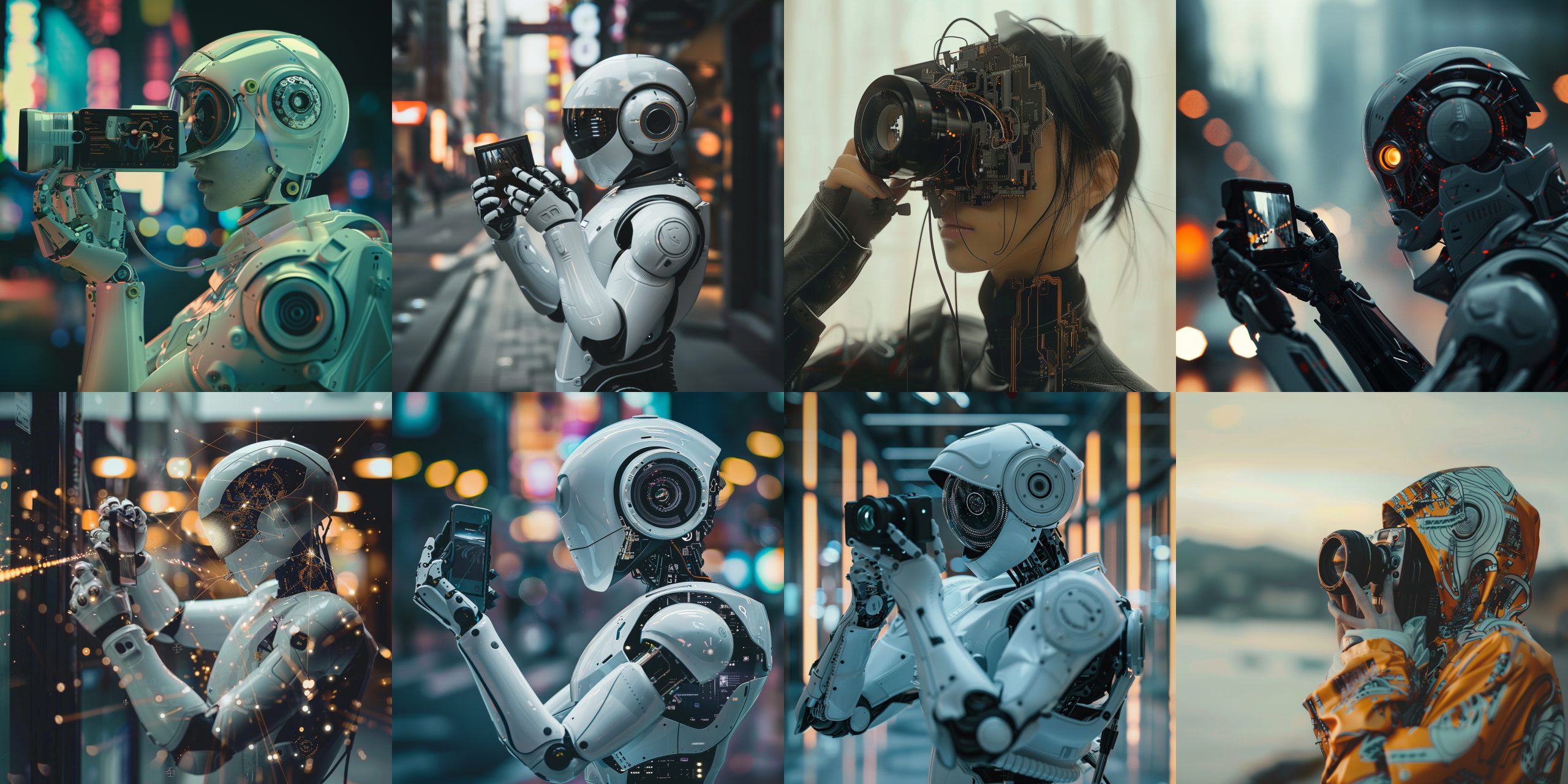
I am sure you will by now have seen multiple excited headlines about AI image generation. You may also have used at least one of the now numerous publicly accessible AI image generation tools. But what does this new, and undoubtedly exciting, potential for imagery mean for the visualisation of climate change? Note: this piece contains images that, whilst generated by AI, may be distressing to some viewers.
Over the last two years, text-to-image AI models such as OpenAi’s DALL-E, Midjourney and Microsoft Bing’s Image Creator tools have exploded in popularity. The tools are capable of generating images from text prompts inputted by a user. These prompts can be anything from simple descriptors, to complex instructions on content and style. When the technology first emerged, the images generated were heavily stylized, often fairly obvious ‘AI images’. Most were interesting for their combination of technical excitement and humour. However, as the technology has developed, the quality of the imagery has dramatically improved. We are now at a point where AI generated images can convincingly pass as photographs, including photojournalistic images. In our ever-increasingly visual media environment this poses a new and developing dilemma for photography producers and consumers.
Climate Visuals’ evidence base stresses the importance of authenticity in photographs of climate change. Our guidance highlights the need to show real people, to avoid using the same familiar visual metaphors and the risks of an over-reliance on staged imagery and protest photographs. Polar bears clinging to ice is a common example of a visual cliche in climate change photography. We know that whilst these familiar images can be effective in signalling to the viewer that a story is about climate change, this might not be a story that they want to read. They are hard to relate to and miss the opportunity for deeper understanding. Audiences also respond poorly to staged photo-opportunities, particularly those involving politicians, and the use of protest imagery can be very divisive. Instead of visual cliches, photographs should communicate new, relatable and detailed stories about real people. When tasked with generating “the best image of climate change", an early version of MidJourney AI’s image generator produced these four images:
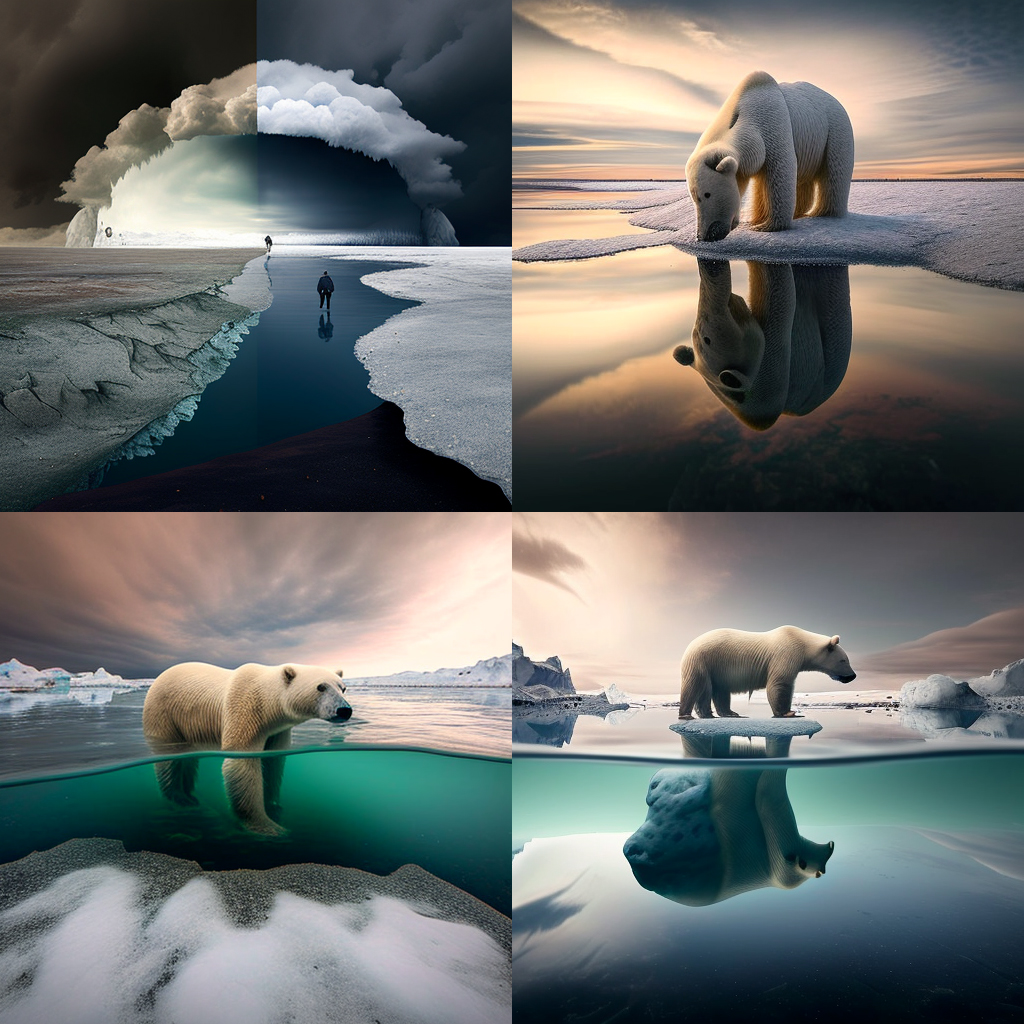
The top left frame appears to be an interpretation of a photomontage, and is quite visibly a non-real image. However the other three, whilst having a number of visual elements that help to identify them as not photographs (for example the ice in the reflection of the bear in the bottom-right image), are clearly mimicking the classic visual stereotype of a sad polar bear on melting ice. The issue here is not just that the polar bear is not a real one. More, that the AI model has interpreted the prompt with a highly cliched output, a visual representation that we know from our research prompts cynicism and fatigue in audiences. Whilst the images are of interest by virtue of their generation (the medium) they are examples of known ineffective visual representations of climate change (the message). This, even aside from the fact that the bear is not real, should be a guiding reason to avoid using them.
Polar bear images are a familiar example of visual cliches and stereotyping in climate change photography. However users should be aware of the potential for AI generated images to perpetuate visual stereotypes in much more subtle, but significant ways. When asked to generate “an award winning documentary photograph of climate change” Midjourney’s AI delivered these four images:

Similarly when asked for “climate change photojournalism” these images were generated:

In both of these examples the images generated mimic a number of classic visual stereotypes - lone victims in the face of catastrophic climate change impacts, an overwhelming sense of disaster, destruction and death. The images are hopeless and devastating. If we consider the images as photographs, the people featured lack any agency in their fate, they are powerless, anonymous and presented only for the sympathy of the viewer. Significantly, often the images appear to depict non-white figures as victims. In contrast, responding to the prompt “photograph of a climate scientist at work” the images generated often feature white figures:

A major concern generally with AI generated images is that we don’t know what images they are trained on. We therefore don’t know what representations of climate change the models are drawing on to generate new images, or how this material is interpreted. Anecdotally it appears that AI generated images will often repeat common visual representations. Whilst the images may be new, the content regularly appears to be an imitation of visual cliches and has an overreliance on damaging, ethically troubling visual stereotypes. With photography we must move away from these stereotypes, such as those perpetuating victim narratives, and instead centre dignity and ethical storytelling and prioritise diversifying those behind, and in front of, the lens. With AI generated images now having the potential to be used in place of photographs, it is vital to consider them as critically as photography.
Central to the Climate Visuals guidance is the need to show real people and tell real stories in images of climate change, be it in relation to causes, impacts, or solutions. Before the emergence of generative AI images this would have been interpreted to mean avoiding staged photocalls, including those of politicians, and images that are clearly staged for the purposes of a photograph. There has always been some nuance to this; not all ‘stock’ photography as illustration is bad by default, but with the development of AI image generation this takes on a new significance. There is no depth to the story of an AI image, no detailed story or narrative for a viewer to engage with and hopefully relate to. Photography has the potential to communicate detailed, complex narratives, particularly in photojournalism. Images generated by AI are immediately reduced to purely illustrative, surface level, content. We know from our evidence base that images of real people are favoured by audiences. We also know viewers shouldn’t be underestimated in their ability to see through inauthentic, staged images. We need to prioritise telling real, diverse, stories that are relatable to wide ranging audiences - AI generated images ignore the potential of this photographic storytelling and instead settle for surface level illustrations not grounded in real experience. As AI generated images become increasingly realistic, the need for transparency and honesty in their use is paramount. We have already seen the damaging potential of political AI generated images, as well as the debate around these images posted, and then deleted, by Amnesty International. If AI-generated images are used in the context of real events and presented as photographs, reality or without obvious labelling, then their use moves into the territory of being actively misleading to audiences. The images contain no reality, and should be considered more akin to artist representations, of which the creator has minimal input control, than photography.
Away from the ethical and moral issues of using AI generated images, there is also the potential for legal issues. As a developing technology the legal framework surrounding generative AI imagery is incomplete. The copyright status of images generated by a variety of AI tools are subject to multiple court cases globally. Similarly, there is a significant debate around the legality and fair-compensation of those whose work has been used to train the AI models. The images and data used to train the models are almost entirely unknown, and any compensation to those whose creative works have been used in this way is mostly non-existent. Academics have also found that it is easily possible to generate plagiaristic outputs with AI tools. In one major case, Getty Images, one of the largest imagery providers in the world, is currently pursuing legal action against Stability AI (whilst also introducing an AI generation tool itself). The temptation to use AI to generate ‘free’, increasingly high quality images, is clear, but the reality and ethics of this is far from it. As the legal arguments develop, and as more commercial AI image generation tools become available these grey areas will develop clarity, but currently this is an area of significant uncertainty.
Photography can tell compelling, detailed stories, and presents a vital opportunity for real and lasting public engagement. The development of generative AI imagery is undoubtedly a huge moment in how society produces and consumes images. However, the potential issues and pitfalls of using AI images in place of photography must be taken seriously. In visualising climate change through photography we should be seeking to tell real, compelling, relatable stories to our audiences. This is not possible through AI generated images, which construct scenes from unknown input datasets and are more closely related to digital art than photography. As AI generated images become more able to mimic photographs, thorough, ethical, photojournalism, comprehensive captioning and detailed and transparent crediting of images becomes ever more urgent and essential.